Quantitative Data Analysis
Very specific, technical procedures.
Not necessary if you are looking at qualitative data.
Descriptive statistics, but bivariate and multivariate required to measure strengths of associations. Most commonly used application is SPSS.
Must test if there is a significant difference that is statistical. Probability value given p=0.1 means 1 percent chance therefore considered significant.
Regression Models:
Linear or multiple variables.
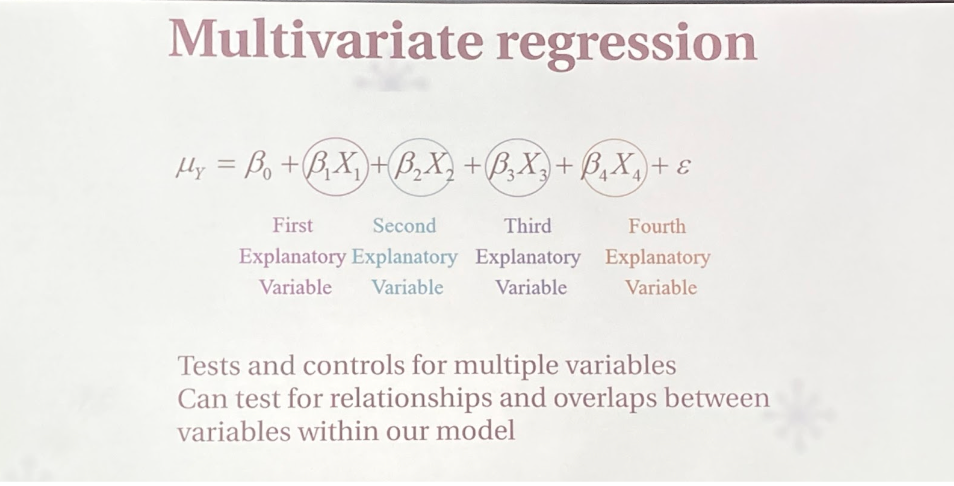
Caveats:
Correlation/significance does not necessarily mean causation.
Qualitative Coding and Thematic Analysis
Far more subjective than quantitative.
Aim is to try and help us to understand the data in front of us and bring to the surface the patterns in our data. Core aim is not to be anecdotal.
Usually leads to large, unwieldy datasets. Allows for inductive analysis and building of theory.
Should remain faithful to the data. Analysis grounded by the data.
Hints:
Familiarity with the data is key.
Make notes filed in a systematic way.
Synopses of interviews are useful.
Transcribe yourself, it helps to get familiar with the data.
Thematic coding:
Assigning labels to data based on patterns we have identified.
Code = a word or short phrase that captures a summative, salient, essence Saldana, 2008. Capturing. Is an evocative attribute of a portion of data.
Open coding
Can be delimited by RQs, but can also inform new theories.
Needs hours at a time of highly functioning critical thought. Keep a methodical record of your codes. Keep a record of where the codes appear. Keep a record how the codes have appeared.
Once done, then group into larger generalised groups.
Create an index that sorts codes and categories.Then apply numbers from the index to the data.
Software available = Nvivo, atlas.ti
This kind of data analysis is not looking for frequency of words.
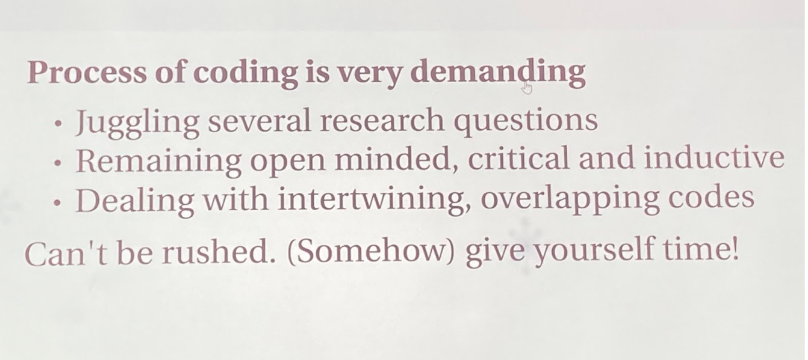

Content & Discourse Analysis
Content Analysis:
Involves analysis of documents, texts or visual material.
Transforms qualitative data into quantitative data, aim to be objective and systematic. RESEARCH IS REPLICABLE.
Good for analysis of newspaper articles, tweets, tv programmes etc.
Highly specific rules for coding required. Personal bias is removed due to the rules established.
Codes are dependant on RQs
Pros:
Replicable, longitudinal, unobtrusive, highly flexible, long term sampling.
Cons
Hostage to quality of data
Interpretive element = bias, meaning is absent, measurement and not understanding.
Discourse Analysis:
Form of qualitative research.
Notion of discourse pioneered by Foucoult, similar to Kuhn’s notion.
Discourses frame our comprehension, our identities and intelligible questions.
See also CRITICAL DISCOURSE analysis.
Emancipatory/liberato
ry approach. Political agenda.
All in the text.
Tend to analyse small volumes of data through a very fin grained approach. Most commonly textual data.
SIMPLY
What discourse is doing
How the discourse is constructed to make this happen. (Bryman, 2008, drawing on Potter).
Linguistic in its approach, structure of text grammatical and semantic. Collocations and use of metaphors (Taylor).
Aiello and Parry talk about the critical discourse of the imagery.
DA is a craft skill, learnt more like an art than a statistical technique (Potter).
DISCOURSE ANALYSIS OF THE FOLLOWING:
National Plan For Music Education
Model Music Curriculum
Analyse the language in the NPfME
Analyse where genres are situated.
Policy Analysis & Systematic Reviews
Policy Analysis:
Atkins and Wallace - an approach in which the researcher analyses national, local or institutional policy. Actual or potential impact on the sector.
Who is involved, processes of implementation.
Rigorous process
Aims, what is the policy trying to do, is there a problem it is trying to solve?
Key stakeholders for the policy? What are they likely to do/think about it?
Design, how well does the design of the policy reflect the aims of the policy?
Is it technically feasible? Cost-effective?
Knock-on effects, where the policy might impact other areas.
Implementation - what are the risks? Knock on effects that have not been considered. What scope is there for evaluation of the policies impact? How will we know whether it has achieved its aims?
Would need to demonstrate a huge amount of knowledge around evaluation.
Assessment requires a literature review.
INCLUDE APPENDICES WITH EXAMPLES OF WHAT YOU”VE DONE
Systematic Reviews:
Replicable, scientific, transparent.
Systematic and unbiased.
Comprehensive account and review of the existing evidence.
Generic steps (Millar)
Aim
Inclusion and exclusion criteria to guide the studies
Sample
Record key features
Synthesise results
Meta-analysis - statistical treatment of studies sampled. Summary tables.
If the output of the review is small, we could conduct qualitative analysis.
Narrative, arts-based and participatory research
Rosa Marvell
Aligns with interpretivism.
Create collaborative,caring, empathetic research experiences.
Embedded power imbalances within the education sector.
Graphic Timelines:
Temporal
Narrative interviews:
Personal meaning-making
Focuses on what’s meaningful for the participant
Nice to have conversation
Photo elicitation vs photo voice
Elicitation = interview prompts, produced by researchers or participants, facilitating role of researcher
Photo voice = action research, participant driven, political aims?
Mobile methodologies:
Interaction - then interview
Following objects
Participatory/ action research
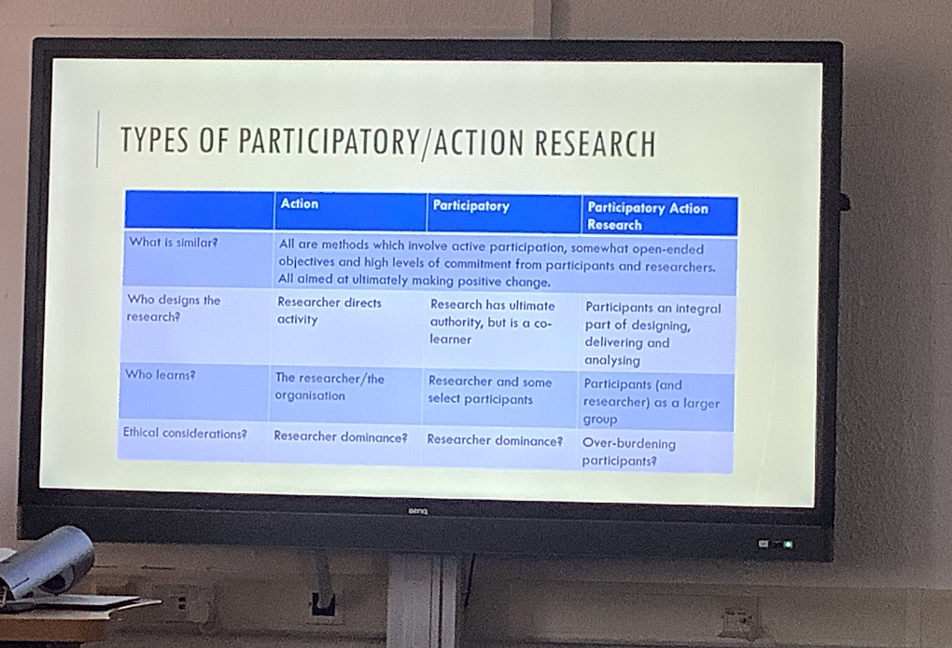
Comments